考試分值占比 20%(無變化)。
考綱考點(diǎn)新增 Machine learning(機(jī)器學(xué)習(xí));第七章加入考點(diǎn):Estimate the correlation coefficient from the R2 measure obtained in linear regressions with a single explanatory variable(用一元線性回歸的 R 方測(cè)度來估計(jì)相關(guān)系數(shù));
第八章加入考點(diǎn):Calculate the regression R2 using the three components of the decomposed variation of the dependent variable data: the explained sum of squares, the total sum of squares, and the residual sum of squares(使用解釋變量數(shù)據(jù)中分解變動(dòng)的三個(gè)分量:ESS、TSS、RSS 計(jì)算回歸的 R 方);第十二章加入考點(diǎn):Compare and contrast the different measures of correlation used to assess dependence(比較和對(duì)比用于評(píng)估依賴性的不同相關(guān)性測(cè)度).
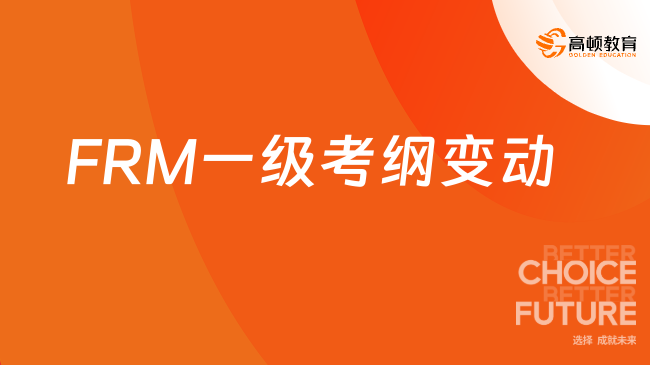
考試分值占比 30%(無變化)。
僅對(duì)一處內(nèi)容做了刪減:將“Describe delta hedging for options as well as for forward and futures contracts”改為“Describe delta hedging for an option.”
考點(diǎn):
金融機(jī)構(gòu)的結(jié)構(gòu)與功能,場(chǎng)外交易(OTC)和交易所市場(chǎng)的結(jié)構(gòu)和機(jī)制,遠(yuǎn)期、期貨、互換和期權(quán)的結(jié)構(gòu)、機(jī)制和估值,使用衍生品進(jìn)行對(duì)沖,匯率與外匯風(fēng)險(xiǎn),期權(quán)估值
考試分值占比 30%(無變化)。
僅對(duì)一處內(nèi)容做了刪減:將“Apply the exponentially weighted moving average (EWMA) approach and the GARCH (1,1) model to estimate volatility, and describe alternative approaches to weighting historical return data.(應(yīng)用指數(shù)加權(quán)移動(dòng)平均(EWMA)方法和GARCH(1,1)模型來估計(jì)波動(dòng)率,并描述加權(quán)歷史回報(bào)數(shù)據(jù)的替代方法)”改為“Apply
the exponentially weighted moving average (EWMA) approach to estimate volatility, and describe alternative approaches to weighting historical return data.(應(yīng)用指數(shù)加權(quán)移動(dòng)平均(EWMA)方法來估計(jì)波動(dòng)率,并描述加權(quán)歷史回報(bào)數(shù)據(jù)的替代方法)”.
考點(diǎn):
公司債券,抵押擔(dān)保債券,固定收益估值,利率與利率敏感性度量指標(biāo),對(duì)沖,在險(xiǎn)價(jià)值(VaR),預(yù)期損失(ES),估計(jì)波動(dòng)性和相關(guān)性,經(jīng)濟(jì)資本與監(jiān)管資本,壓力測(cè)試和情景分析,國家和主權(quán)風(fēng)險(xiǎn)模型及風(fēng)險(xiǎn)管理,外部和內(nèi)部信用評(píng)級(jí),預(yù)期與非預(yù)期損失,操作風(fēng)險(xiǎn)。
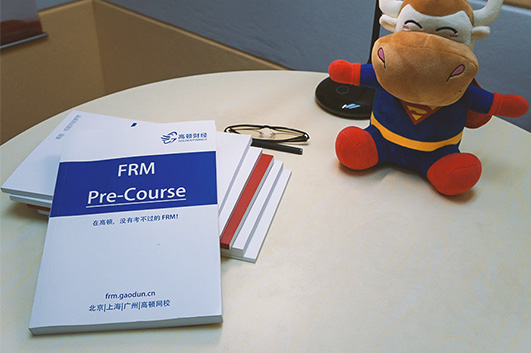